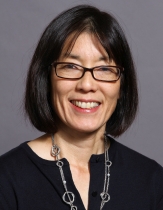
Jody Tanabe
Parkinson disease (PD) is a common neurodegenerative disease that affects 6 to 10 million people worldwide, incurred an estimated $14.4 billion dollars in medical costs alone in the United States in 2010, and is projected to become a greater burden as the population ages.1 The cardinal signs of PD are bradykinesia, tremor, rigidity, and postural instability. The degenerative process leads to the destruction of dopaminergic neurons in the substantia nigra pars compacta (SNc) that project to the striatum. Neuronal loss and the presence of Lewy bodies in the SN and locus coeruleus (LC) provide a histopathologic diagnosis of PD, though the implications of Lewy bodies outside the SN or LC are uncertain.2
On imaging, narrowing of the SNc on T2-weighted images may be seen, but the finding is highly variable, and the main role of MRI is to exclude other etiologies (ie, neoplasm, vascular, etc) or support the diagnosis of atypical forms of parkinsonism, including multisystem atrophy (MSA) and progressive supranuclear palsy (PSP). The “hummingbird sign” in PSP or the “hot-cross bun sign,” T2 lateral putaminal hyperintensity, and brain stem atrophy in MSA are useful radiologic findings suggesting atypical parkinsonism. Distinguishing idiopathic PD from atypical parkinsonism is important, as treatments and prognoses differ. In light of the fact that idiopathic PD is by far the most common cause of parkinsonism, however, the current role of diagnostic imaging is limited.
While the classic features of PD revolve around debilitating motor symptoms, a substantial percentage of patients with PD have cognitive deficits, with up to 75% of those with PD developing dementia after 8 years,3 possibly rising to 83% at 20 years.4 The mechanisms of cognitive decline are unknown, though there is an association between the presence of intraneuronal Lewy bodies and cognitive impairment. A previous study showed that cognitive decline correlated with regional Lewy body density predominantly in the anterior cingulate and entorhinal cortex.5 Compared with healthy controls, patients with PD with mild cognitive impairment have lower D2 dopamine receptor levels in the associative (ie, cognitive) striatum as well as the insular cortex, suggesting coupling between striatal and limbic systems.6 The hippocampus has also been implicated as a mediator of cognitive dysfunction in PD, based on preclinical and in vivo volumetric and functional work in PD.7 These studies suggest that neuropsychological features of PD may be understood as circuit pathologies, involving limbic, executive function, or memory pathways, and consistent with parallel cortico-striato-pallido-thalamic-cortico circuit models of the disease.8
High-frequency deep brain stimulation (DBS) is used increasingly to treat medically refractory tremors or treatment-related complications such as dyskinesias in PD. The subthalamic nucleus (STN), located between the red nucleus medially and the SN laterally; internal pallidum; and thalamus are potential targets, associated with different degrees of efficacy and side effects. Efficacy rate as measured by percent of patients with off-period reduced or abolished tremor has been reported to be 54% for STN and 33% for pallidal DBS. Efficacy for dyskinesia is approximately 75% for STN and 65% for pallidal DBS.9 Although DBS was first described in the 1980s,10 the technique is still relatively novel and mechanisms of action are not well understood. Functional and structural imaging, in conjunction with neural modeling, electrophysiologic recordings, and better clinical characterization will improve our ability to predict efficacy and adverse side effects associated with DBS.11
The current AJNR News Digest focuses on advanced imaging to optimize anatomic delineation of midbrain structures that are etiologically involved in PD,12 distinguish idiopathic PD from atypical parkinsonism,13,14 investigate pathway disruptions as possible mechanisms of cognitive impairment,15,16 and optimize coregistration methods to improve DBS targeting.17
Delineating midbrain anatomy could lead to studies that will improve our understanding of both motor and nonmotor aspects in PD, but anatomic detail is limited at clinical field strengths. Gore et al investigated midbrain dopaminergic subcortical structures in healthy controls at 7T. Qualitative assessment, contrast-to-noise ratio (CNR) and volumes of the ventral tegmental area, SN, and red nucleus were studied. They compared a mixed T2- and T2*-weighted gradient-echo (GRASE) to a T2*-weighted fast field echo (FFE) sequence. Both GRASE and FFE clearly delineated the midbrain anatomy. Compared with FFE, GRASE better distinguished densely versus poorly vascularized subregions of the red nucleus. As expected, CNR was higher at 7T compared with values previously reported at 4T. Although the distinction between SN pars compacta (SNc) and pars reticulata (SNr) at 7T was judged to be good, the authors note that reliable boundaries between SNc and SNr have yet to be established. Future studies applying these high-field metrics in patients with PD are needed to determine the utility of 7T MRI.
Differentiating idiopathic PD from atypical parkinsonian syndromes such as MSA and PSP can be difficult. Qualitatively, basal ganglia hypointensity and linear putaminal hyperintensity on T2-weighted images suggest the diagnosis of MSA, and further separation into MSA Parkinson (MSA-P) and cerebellar (MSA-C) subtypes is a goal for diagnosis and treatment. Focke et al extend this by quantifying R2, R2*, DTI, and magnetization transfer parameters in patients with PD, MSA-P, MSA-C, PSP, and in healthy controls.13 Pallidal and putaminal iron content, as measured with R2*, differentiated MSA-P from PD and healthy controls with high predictive power. There was a small but significant increase in mean diffusivity in the SN of PSP compared with the other groups. Overall, however, R2, DTI, and MT measures were relatively insensitive to group differences. Strengths of this study included its quantitative approach, prospective design, and rigorous statistical approach. However, due to small samples sizes (9 PSP, 10 MSA-P, 12 idiopathic Parkinson syndrome, 13 controls) these interesting results will have to be replicated in larger studies.
As an alternative to studying PD disease-related DTI at the group level, Haller et al used machine learning to classify patients at the individual level. Support vector machine (SVM) analysis of imaging data fundamentally differs from the more common group-level voxel-wise analyses. Voxel-wise analyses are mass-univariate tests that separately analyze each voxel across 2 or more groups. SVM is a multivariate analysis of patterns across voxels, resulting in each individual having one pattern, with the goal of identifying patterns that allow discrimination between individuals. Haller et al retrospectively investigated 17 patients with PD and