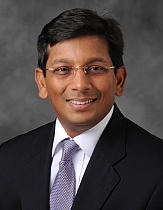
Rajan Jain
Recent improvements in determining molecular/genomic expression of any tissue have brought us one step closer to personalized treatment for many disease processes, notably in oncology. This could be especially true for glioblastomas as the currently available treatment options have not improved the dismal survival. One of the major reasons for this is that current treatment approaches don’t have sufficiently specific molecular targets. Characterization of glioblastoma multiforme remains challenging because the tumors are heterogeneous pathologically and genetically. Meanwhile, noninvasive in vivo quantitative imaging parameters such as perfusion parameters have achieved better integration and routine use, particularly in oncologic imaging, as diagnostic, predictive, and prognostic tools. Many of these imaging parameters, with improvements in technology and standardization, are on the cusp of being promoted to the status of biomarkers. One major step toward this involves the establishment of a specific physiologic and molecular basis for these parameters. The availability of genomic data provides an opportunity to accomplish this and hence a new field is emerging that integrates imaging parameters with molecular/genomic markers. This new field has been called radio-genomics or imaging genomics.
The Cancer Genome Atlas (TCGA) researchers have cataloged recurrent genomic abnormalities in glioblastomas, and in parallel the Cancer Imaging Program is retrospectively obtaining imaging data for TCGA patients and making these available via the Cancer Imaging Archive. These programs provide easy access to genomic and imaging data collected from multiple institutions and hence, foster research opportunities. Our initial success with some very provocative results establishing a genomic basis for perfusion parameters (tumor blood volume and leakiness or permeability) has led us to apply this added knowledge in the clinic. We are currently focusing on integrating the perfusion data with the genomic expression in glioblastomas and using the combined information as a prognostic tool (Jain et al. Genomic mapping and survival prediction in glioblastoma: Molecular sub-