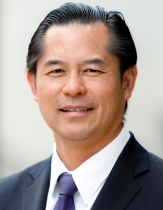
Meng Law
Dynamic susceptibility contrast perfusion MR imaging has been extensively investigated in brain diseases because cerebral perfusion and, more recently, the brain oxygen extraction/consumption are affected in many neurological disorders ranging from gliomas to autoimmune/inflammatory diseases (eg, multiple sclerosis) to neurodegenerative diseases (eg, Alzheimher disease). We chose to determine if there were more optimal methods for characterization and quantitation of relative cerebral blood volume (rCBV). Traditionally in the clinic, we have been limited to operator dependent region of interest (ROI) analysis of perfusion, DTI, and other quantitative MRI metrics.
Histogram analysis was one simple step that would be utilized in clinical practice to improve our reproducibility and also help in our quest toward more standardized reproducible quantitative imaging. Several of the key challenges we face in neuroimaging now include standardization on the acquisition side, optimization on the analysis/image processing side, and developing new quantitation methods to increase our specificity in neurodiagnoses. We had hoped that by investigating whether histogram analysis can be used to quantify rCBV we could begin to move toward more automated, perhaps even statistics-based, methods such as voxel-based morphometry (VBM), and then even more complex machine learning methodologies, by involving multiple quantitative imaging metrics in an algorithmic approach.
In our own practice, we are developing more automated, reproducible methods for quantitation of MRI metrics, but as with any measurement tool (even something as simple as weighing oneself on the bathroom scale each morning), there is variability, standard deviation, and inherent error in our measurements. Further work is being done by members of our current