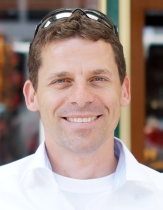
Sven Haller
I chose this topic because I am convinced that many neurodegenerative diseases have specific patterns of brain alterations that are too subtle to be detected by visual inspection alone, particularly at early stages of the disease. Early diagnosis is essential in order to initiate treatments to stop or at least slow down future neurodegeneration. This requires early detection at the individual level, which can be obtained using pattern recognition approaches known from machine learning.
Note that such pattern recognition analyses represent a fundamental shift in paradigm. Classic advanced neuroimaging studies compare a group of patients with a group of controls to identify brain areas involved in a given disease. Such results are fascinating from a research perspective, yet the results cannot be transferred to the diagnosis of individual patients. Pattern recognition analyses, in contrast, allow for detection of individual patients. It is fundamental to highlight that the clinically relevant question of our manuscript is the discrimination of typical PD versus atypical PD, which might be difficult on clinical grounds, in particular at early stages of the disease process. This in turn implies that pattern recognition studies should not compare patients versus controls, as this is not a clinically relevant question and is already possible based on clinical grounds. AJNR was visionary enough to detect this shift in paradigm and accept our study, which compares typical PD versus atypical PD, ie, the clinically relevant distinction, despite the fact that there is no healthy control group as would be required in “classic” group-level comparison studies.
There are, in my opinion, 2 main issues that remain to be solved prior to application of pattern recognition approaches in clinical practice. The first issue is methodologic. Pattern recognition approaches may detect very subtle brain alterations, which are well in the range of inter-scanner variability. This requires strict standardization of MR acquisitions—for example, using dedicated phantoms such that the dataset of an individual patient acquired on one MRI machine can be analyzed by a classifier trained and validated in a dataset of patients acquired on another (or ideally many other) MRI machine. The second issue is medico-legal. It is obvious that no technique may be 100% correct. Alterations detected using pattern recognition are complex and subtle, and even an experienced radiologist may not necessarily be able to validate these alterations. Therefore, the question arises how false-positive and false-negative results by the automatic classifier should be treated from a medico-legal perspective.
I have received excellent feedback so far. An increasing number of clinicians and researchers recognize that individual-level pattern recognition analyses will eventually allow early and specific diagnosis of individual patients. A related study in the domain of mild cognitive impairment1 won the Lucien Appel Prize by the European Society of Neuroradiology in 2011.