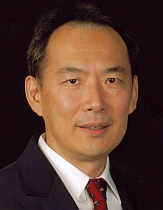
Osamu Sakai
We are interested in investigating postprocessing techniques that could potentially be used as an adjuvant diagnostic aid in clinical practice. Recently, we have been exploring the use of image texture analysis to help quantify subtle changes in image patterns within a tumor volume to potentially predict diagnostic and prognostic features of the tumor.1
Image texture is defined as “a complex visual pattern within an image that consists of simpler sub-patterns with characteristic features which may be evaluated through quantitative analysis.” Texture analysis is a branch of image processing that seeks to reduce image information by extracting texture descriptors from the image, which may allow for the mathematical detection of subtle changes among image pixels.2,3
Texture analysis has been in use for many years in the analysis of satellite images for military reconnaissance, photographs for quantitative mapping of geographical terrain, and fabrics for fast detection of flaws in the textiles.
Within the field of radiology, the use of a texture analysis is relatively new and is being explored in evaluating subtle pathologic changes in an image, which are not easily quantifiable by the human eye. It has been used for the evaluation of brain parenchyma in patients with seizure,4 microfractures in bone, cartilage integrity,5 and the quantification of hepatic fibrosis.6,7
There are 2 important aspects to the use of a texture analysis in the field of radiology: 1) quantification of visible findings and 2) detection and quantification of findings invisible to the human eye. Quantitative evaluation using texture analysis may allow us to perform more scientific assessment of images that have only been evaluated qualitatively. Texture analysis is a postprocessing technique and does not require purchase of expensive hardware or devices.